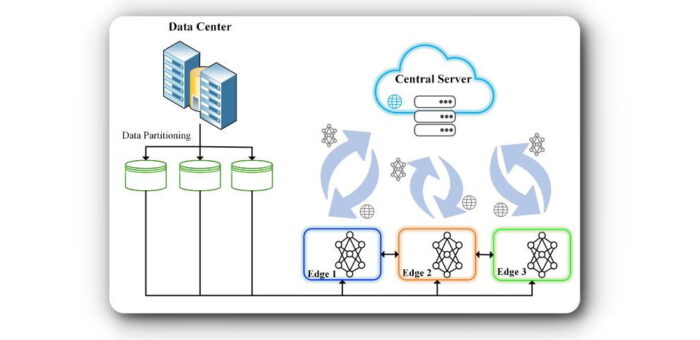
Article “Distributed Learning Applications in Power Systems: Methods, Gaps, and Challenges” has been published in MDPI Energies
Article "Distributed Learning Applications in Power Systems: Methods, Gaps, and Challenges" has been published in MDPI Energies. Congratulations, Nastaran! Abstract: In recent years, machine learning methods have found numerous applications in power systems for load forecasting, voltage control, power quality monitoring, anomaly detection, etc. Distributed learning is a subfield of machine learning and a descendant of the multi-agent systems field. Distributed learning is a collaboratively decentralized machine learning algorithm designed to handle large data sizes, solve complex learning problems, and increase privacy. Moreover, it can reduce the risk of a single point of failure compared to fully centralized approaches and lower the bandwidth and central storage requirements. This paper introduces three existing distributed learning frameworks and reviews the applications that have been proposed for them in power systems so far.…